Deep Learning Convolutional Neural Network applying for the Arctic Acoustic Tomography Current Inversion Accuracy Improvement.
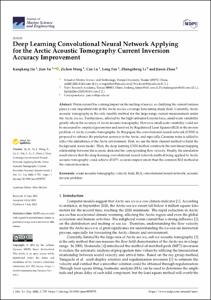
View/ Open
Average rating
votes
Date
2021Author
Jin, Kangkang
Xu, Jian
Wang, Zichen
Lu, Can
Fan, Long
Li, Zhongzheng
Zhou, Jiaxin
Metadata
Show full item recordAbstract
Warm current has a strong impact on the melting of sea ice, so clarifying the current features plays a very important role in the Arctic sea ice coverage forecasting study field. Currently, Arctic acoustic tomography is the only feasible method for the large-range current measurement under the Arctic sea ice. Furthermore, affected by the high latitudes Coriolis force, small-scale variability greatly affects the accuracy of Arctic acoustic tomography. However, small-scale variability could not be measured by empirical parameters and resolved by Regularized Least Squares (RLS) in the inverse problem of Arctic acoustic tomography. In this paper, the convolutional neural network (CNN) is proposed to enhance the prediction accuracy in the Arctic, and especially, Gaussian noise is added to reflect the disturbance of the Arctic environment. First, we use the finite element method to build the background ocean model. Then, the deep learning CNN method constructs the non-linear mapping relation.....
Resource URL
https://www.mdpi.com/2077-1312/9/7/755Journal
Journal of Marine Science and EngineeringVolume
9Issue
Article 755Page Range
15pp.Document Language
enSustainable Development Goals (SDG)
14.aMaturity Level
Pilot or DemonstratedSpatial Coverage
Arctic RegionDOI Original
https://doi.org/10.3390/jmse9070755Citation
Jin, K., Xu, J., Wang, Z., Lu, C., Fan, L., Li, Z. and Zhou, J. (2021) Deep Learning Convolutional Neural Network applying for the Arctic Acoustic Tomography Current Inversion Accuracy Improvement. Journal of Marine Science and Engineering, 9:755, 15pp. DOI: https://doi.org/10.3390/jmse9070755Collections
- CAPARDUS Practices [147]
The following license files are associated with this item: